Figure 2A
First compute for shape parameter \(a=0.248\), \(a=1\), \(a=2\) and \(a=15\) the respective scale parameter \(b\) such that the overall connection probability equals \(\mu = 0.1\).
Then we get the relative occurrence of bidirectionally connected pairs \(\varrho\) via
The probability density function fT
was defined gamma_functions
and is explained in the previous section.
import pylab as pl import numpy as np from scipy.optimize import brentq import scipy.integrate as integrate from scipy.stats import gamma from gamma_functions import K, fT # for different values of a, determine b such that \mu = 0.1 mu = 0.1 def root_f(b): return integrate.quad(lambda x: x*fT(x,a,b),0,1)[0] - mu a_ns = [0.248,1.,2.,15.] b_ns = [brentq(root_f, 0.5*mu/a, 5*mu/a) for a in a_ns] # for a,b pairing get the relative overrepresentation \rho def get_rho(a,b): numer = integrate.quad(lambda x:x**2*fT(x,a,b),0,1)[0] denom = (integrate.quad(lambda x: x*fT(x,a,b), 0,1)[0])**2 return numer/denom rhos = [get_rho(a,b) for a,b in zip(a_ns, b_ns)] print "a_ns: ", a_ns print "b_ns: ", b_ns print "rhos: ", rhos
With this we can create the graphic
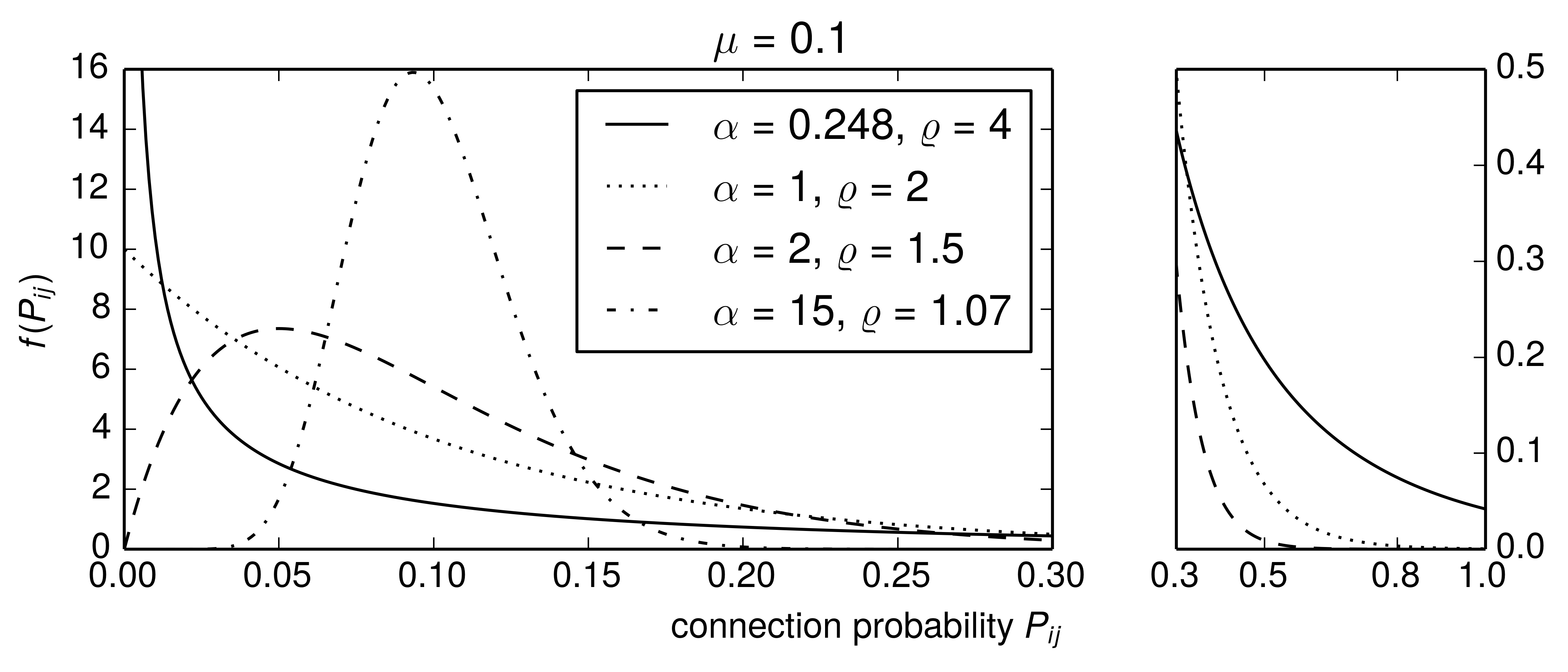
by plotting the probability density functions for the \(a,b, \varrho\) pairings. We can use gamma.pdf
from the scipy.stats
and only have to scale it by K(a,b)
to get the density function for the truncated version.
from matplotlib import rc from matplotlib import gridspec rc('text', usetex=True) pl.rcParams['text.latex.preamble'] = [ r'\usepackage{tgheros}', # helvetica font r'\usepackage{sansmath}', # math-font matching helvetica r'\sansmath' # actually tell tex to use it! r'\usepackage{siunitx}', # micro symbols r'\sisetup{detect-all}', # force siunitx to use the fonts ] fig, ax = pl.subplots(1,1) fig.set_size_inches(8.2,2.8) fig.suptitle(r'$\mu = 0.1$', fontsize=15) gs = gridspec.GridSpec(1,2, width_ratios = [3,1]) ax_left = pl.subplot(gs[0]) ax_right = pl.subplot(gs[1]) x = np.arange(0,1.+0.001, 0.001) linestyles = ['-',':','--','-.'] for a,b,rho,ls in zip(a_ns, b_ns,rhos,linestyles): # quick sanity check h = integrate.quad(lambda z: K(a,b)*gamma.pdf(z, a, scale=b),0,1.)[0] assert abs(1.-h) < 10e-6 ax_left.plot(x, K(a,b)*gamma.pdf(x, a, scale=b), 'k', label=r'$\alpha = %g$, $\varrho = %.3g$' %(a, rho), linestyle=ls) ax_left.set_xlim(0,0.3) ax_left.set_ylim(0,16) ax_left.legend() label = ax_left.set_xlabel(r'connection probability $P_{ij}$') ax_left.xaxis.set_label_coords(0.8, -0.135) ax_left.set_ylabel(r'$f(P_{ij})$') for a,b,rho,ls in zip(a_ns, b_ns,rhos,linestyles): ax_right.plot(x, gamma.pdf(x, a, scale=b), 'k', linestyle=ls) ax_right.set_xlim(0.3,1.0) ax_right.set_ylim(0,0.5) ax_right.set_xticks([0.3, 0.5, 0.8, 1.0]) ax_right.set_yticks([0., 0.1, 0.2, 0.3,0.4, 0.5]) ax_right.yaxis.tick_right() pl.savefig('gamma_figure_A.pdf', dpi=600, bbox_inches='tight')